Artificial Intelligence in Healthcare: Legal and Ethical Implications
Artificial Intelligence (AI) is rapidly transforming and playing an increasingly prominent role in a multitude of industries, including the health care field. AI is a computer system developed to perform various tasks or reasoning processes by simulating human cognitive functions through machine learning algorithms [1]. AI technologies are employed to facilitate the responsibilities of health care workers by assisting with tasks that rely on the manipulation of data and knowledge. The application of AI in health care can be examined through two branches: Virtual and physical [2]. The virtual aspect plays an integral role in medical diagnosis and outcome prediction, personalized treatment plans, patient engagement, and health administration applications [3][4]. The physical component of AI utilizes robotics to assist in performing surgeries and other deliveries of care [2]. These AI tools can assist physicians in reducing human errors, making better clinical decisions, and can even surpass their abilities in particular domains such as radiology [2]. The proliferation of AI can create a path towards a more affordable, efficient, and personalized health care system. However, it can also introduce a new set of ethical and legal issues to the health care industry.
AI devices in health care can be dichotomized into two components. The first element employs machine learning techniques that examine structured data such as imaging, genetic, and electrophysiological data to cluster patients with similar traits or prognosticates disease outcomes [3]. The second device uses natural language processing methods that obtain information from unstructured data such as clinical notes and translates them into structured data [3].
Diagnostic errors are a serious threat to health care quality. It is estimated that the rate of outpatient diagnostic errors is at 5.08% in the United States [5]. Advances in image classification have been applied to medical imaging to improve the quality and accuracy of medical diagnosis. Convolutional neural networks (CNNs), a deep-learning algorithm designed for analyzing two-dimensional data such as images, has become a valuable instrument for disease identification and diagnosis [6]. CNN methods have been employed in a broad variety of diseases, including the diagnosis of congenital cataract disease through ocular images, identification of skin cancer from clinical images, detection of referable diabetic retinopathy through retinal fundus photographs, and prediction of malignant lesions and benign lesions and has become fundamental to medical fields that rely on imaging data such as radiology, pathology, and dermatology [3] [6]. Recurrent neural networks (RNNs) are another deep-learning algorithm that processes sequential inputs such as language, speech, and time-series data and is frequently employed in the natural language process for tasks such as machine translation, text generation, and image captioning [7].
IBM Watson, a super-computer that applies both deep learning and natural language processing methods, is the most advanced AI system used in the health care field. IBM Watson is ideal for the health care industry because it enables both clinicians and patients to make informed medical decisions. The super-computer can help mitigate medical errors by providing physicians with prompt answers to any medical questions [8]. IBM Watson, however, is best known for its role in precision care. It analyzes medical records, laboratory results, and clinical research while monitoring the health of the patient throughout the diagnosis and treatment process, providing patients with a personalized, evidence-based treatment [8].
The Electronic Medical Record (EMR) is the primary tool utilized for documenting and sharing medical information. The natural language processing method is employed to analyze unstructured data from the EMR, and IBM Watson is crucial in developing the automated text summarization of EMR data [1] [9]. It is vital to provide an accurate, concise summarization of data for identifying relevant information, as complex, time-consuming data entry can interfere with patient outcomes. IB Watson provides the summarization of EMR data by “identifying key relationships among clinical concepts with a granularity that matches clinical decision making” [9]. EMRs are also being utilized to interpret clinical scenarios via deep-learning methods [7]. Auto-encoders are employed to predict specific diagnoses by modeling the temporal sequence of events that transpired in a patient’s record using CNNs and RNNs [7].
There are several major ethical and legal concerns that could arise out of the increased implication of AI tools. First, there is an ethical concern of biases being incorporated into AI algorithms and devices, which is concerning especially in sensitive domains such as health care. There are also legal issues relating to liability. Since the application of AI in clinical practice is relatively new, there is essentially no case law on liability involving medical AI.
It is important to note that technology is not inherently neutral; they are programmed by fallible human beings who often have their own biases. Health care inequality is closely intertwined with social inequality, as implicit bias in health care workers is significantly related to the interactions, treatment decisions, and health outcomes of a patient [10].
AI algorithms introduced and utilized in nonmedical fields have already been shown to reflect biases inherent in the data used to train them. For instance, in 2014, Amazon built an AI tool to assist with the hiring of employees. However, it was discovered over a year later that the algorithm was discriminating against women. Amazon’s system used the resumés of past candidates submitted to the company over a 10-year period to decide which new candidates were preferable. As a male-dominated tech industry, however, most of the candidates and hires used for input were men. The machine reflected the bias and taught itself to prefer male candidates, penalizing resumes that included the word “women.” [11]
Therefore, similar racial biases could inadvertently be integrated into various health care algorithms, as health care outcomes already vary by race. In many clinical health studies, the research does not accurately reflect the population of interest. They often fail to attain adequate minority representation, especially in cancer clinical trials where there are striking racial disparities in cancer incidence and mortality [12]. Thus, women and minority racial groups generally have poorer treatment options and longitudinal health outcomes [12].
There have already been several incidents of bias in AI algorithms in health care. One example was an auditory test created by Winterlight Labs, to screen for Alzheimer’s disease. The technology was initially published in the Journal of Alzheimer’s Disease where it claimed it boasted a 90% accuracy. However, they realized that their system only worked for Native English speakers of certain Canadian dialects. Errors like this could skew a non-Native English speaker’s result by indicating Alzheimer’s based on their accents or response times [13].
AI in medicine often operates as a “black-box,” meaning humans are unable to conclude how algorithms generate their decisions and outcomes [14]. The lack of transparency in the algorithm makes it is difficult to determine who is liable when a patient is injured through an AI technology. Liability for medical errors falls under tort law, which is a “civil claim in which a party requests damages for injuries caused by a harmful, wrongful act of another [15].” Tort claims in medicine can be categorized into medical malpractice, vicarious liability, and product liability.
Medical Malpractice occurs when a physician fails to meet the professional standards of medicine and subsequently injures a patient [16]. The plaintiff must demonstrate that: 1) the defendant had a duty of care to the plaintiff, 2) defendant failed to conform to the required standard of care either by his acts or failure to act, 3) the plaintiff sustained damages, and 4) the breach of the defendant’s duty was the proximate cause of those damages [16]. However, medical liability becomes more convoluted in clinical practice when AI algorithm is involved.
In general, tort law stresses the standard of care. Under current law, physicians will generally not be held liable as long as they adhere to the standard of care [14]. For example, if an AI algorithm recommends an incorrect standard of care and the physician follows the AI’s recommendation, they will not be held liable despite generating a bad outcome for the patient [14]. However, a physician will face liability when they don’t follow the standard of care and an injury occurs [14].
Vicarious liability holds an individual legally responsible for the acts of another, commonly found in the employer-employee relationship [17]. Therefore, hospitals can be held liable for the acts of their employees, including physicians who commit malpractice [15]. Additionally, hospitals can also be held liable for “failing to exercise due care in hiring, training, or supervising employees, or for failing to maintain adequate facilities and equipment,” such as the actions of their AI algorithms [17].
Under product liability, manufacturers can be found liable for one of three kinds of product defects: manufacturing defects, design defects, and failures to warn [15]. Manufacturing defects occur when a product “does not conform to the manufacturer’s specifications [16].” A product is defectively designed if the condition is unreasonably dangerous for the user or consumer [16]. Finally, the manufacturer is responsible for providing adequate warnings to the consumer of risks inherent in the product [16].
However, the learned intermediary doctrine prevents plaintiffs from seeking recovery and suing the manufactures directly [8]. Under this doctrine, the manufacturer has no duty directly to the patient and the physician becomes a learned intermediary between the manufacturer and the patient [15] To qualify as a learned intermediary, the manufacturer must adequately disclose the risks of the medical device to the patient [8]. The physician is responsible for communicating with their patients about the benefits and dangers of the device and could be held liable if they fail to fulfill their duty [15].
The current legal and ethical standards regarding AI applications in health care is insufficient. The biggest challenge is to the lack of transparency due to the black-box algorithm. While black-box algorithms are extremely helpful for applications in prognostics, diagnostics, image analysis, and personalized treatments, it also makes it particularly hard to examine any possible biases or detect if the algorithmic conclusion is incomplete or inaccurate. Therefore, it is crucial for health care professionals to evaluate the quality and efficiency of black-box algorithms based on procedural measures.
Works Cited
- Miller, D. D., & Brown, E. W. (2018). Artificial intelligence in medical practice: the question to the answer?. The American journal of medicine, 131(2), 129-133.
- Hamet, P., & Tremblay, J. (2017). Artificial intelligence in medicine. Metabolism, 69, S36-S40.
- Jiang, F., Jiang, Y., Zhi, H., Dong, Y., Li, H., Ma, S., … & Wang, Y. (2017). Artificial intelligence in healthcare: past, present and future. Stroke and vascular neurology, 2(4), 230-243.
- Davenport, T., & Kalakota, R. (2019). The potential for artificial intelligence in healthcare. Future Healthcare Journal, 6(2), 94-98.
- Singh, H., Meyer, A. N., & Thomas, E. J. (2014). The frequency of diagnostic errors in outpatient care: estimations from three large observational studies involving US adult populations. BMJ Qual Saf, 23(9), 727-731.
- He, J., Baxter, S. L., Xu, J., Xu, J., Zhou, X., & Zhang, K. (2019). The practical implementation of artificial intelligence technologies in medicine. Nature medicine, 25(1), 30-36.
- Esteva, A., Robicquet, A., Ramsundar, B., Kuleshov, V., DePristo, M., Chou, K., … & Dean, J. (2019). A guide to deep learning in healthcare. Nature medicine, 25(1), 24-29.
- Allain, J. S. (2012). From Jeopardy to Jaundice: The medical liability implications of Dr. Watson and other artificial intelligence systems. La. L. Rev., 73, 1049.
- Devarakonda, M., Zhang, D., Tsou, C. H., & Bornea, M. (2014, October). Problem-oriented patient record summary: an early report on a Watson application. In 2014 IEEE 16th International Conference on e-Health Networking, Applications and Services (Healthcom) (pp. 281-286). IEEE.
- FitzGerald, C., & Hurst, S. (2017). Implicit bias in healthcare professionals: a systematic review. BMC medical ethics, 18(1), 19
- Hamilton, I. A. (2018, October 10). Amazon built an AI tool to hire people but had to shut it down because it was discriminating against women. Retrieved from Business Insider: https://www.businessinsider.com/amazon-built-ai-to-hire-people-discriminated-against-women-2018-10
- Oh, S. S., Galanter, J., Thakur, N., Pino-Yanes, M., Barcelo, N. E., White, M. J., … & Borrell, L. N. (2015). Diversity in clinical and biomedical research: a promise yet to be fulfilled. PLoS medicine, 12(12), e1001918.
- Stanford Medicine. (2018). The Democratization of Health Care. Stanford Medicine 2018 Health Trends Report.
- Price, W. N., Gerke, S., & Cohen, I. G. (2019). Potential Liability for Physicians Using Artificial Intelligence. JAMA.
- Sullivan, H. R., & Schweikart, S. J. (2019). Are current tort liability doctrines adequate for addressing injury caused by AI?. AMA journal of ethics, 21(2), 160-166.
- Chung, J., & Zink, A. (2017). Hey Watson-Can I Sue You for Malpractice-Examining the Liability of Artificial Intelligence in Medicine. Asia Pacific J. Health L. & Ethics, 11, 51.
- Price, W. N. (2017). Artificial Intelligence in Health Care: Applications and Legal Issues. The Scitech Lawyer, 14(1)
PLACE THIS ORDER OR A SIMILAR ORDER WITH ALL NURSING ASSIGNMENTS TODAY AND GET AN AMAZING DISCOUNT

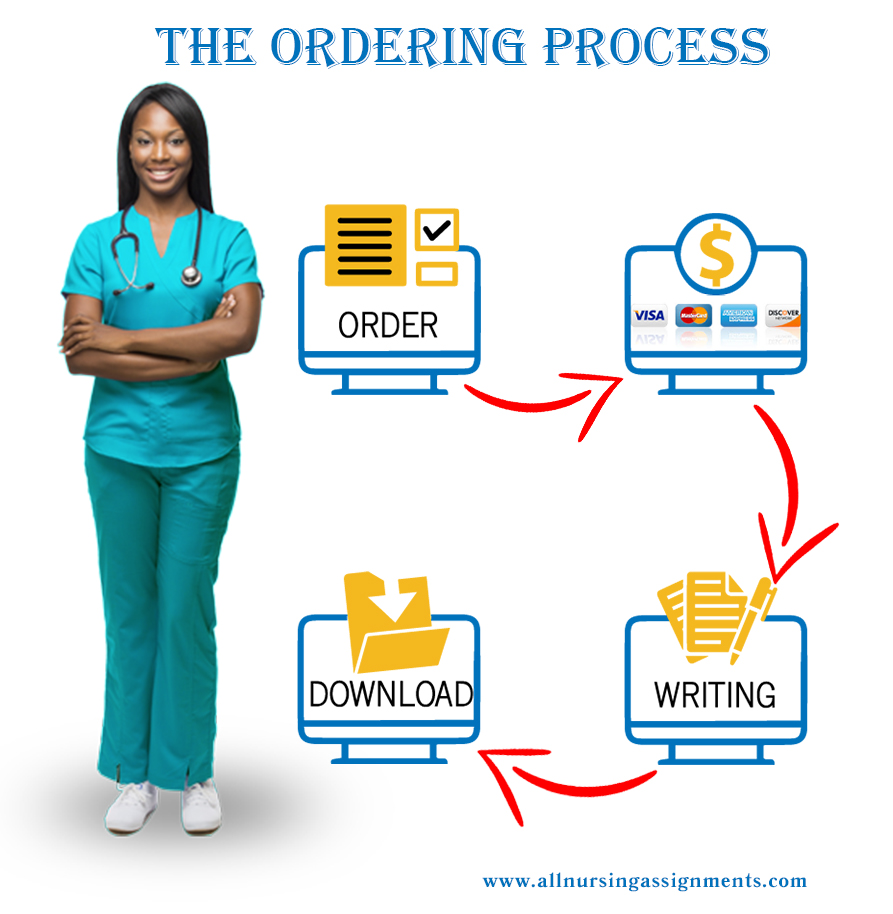